Bayesianism: Constantly Updating to Get Closer to the Truth
Most of us understand statistics through the frequentist model, which relies on large datasets and repeated observations. However, Bayesian thinking offers an alternative approach that continuously updates probability estimates as new evidence emerges.
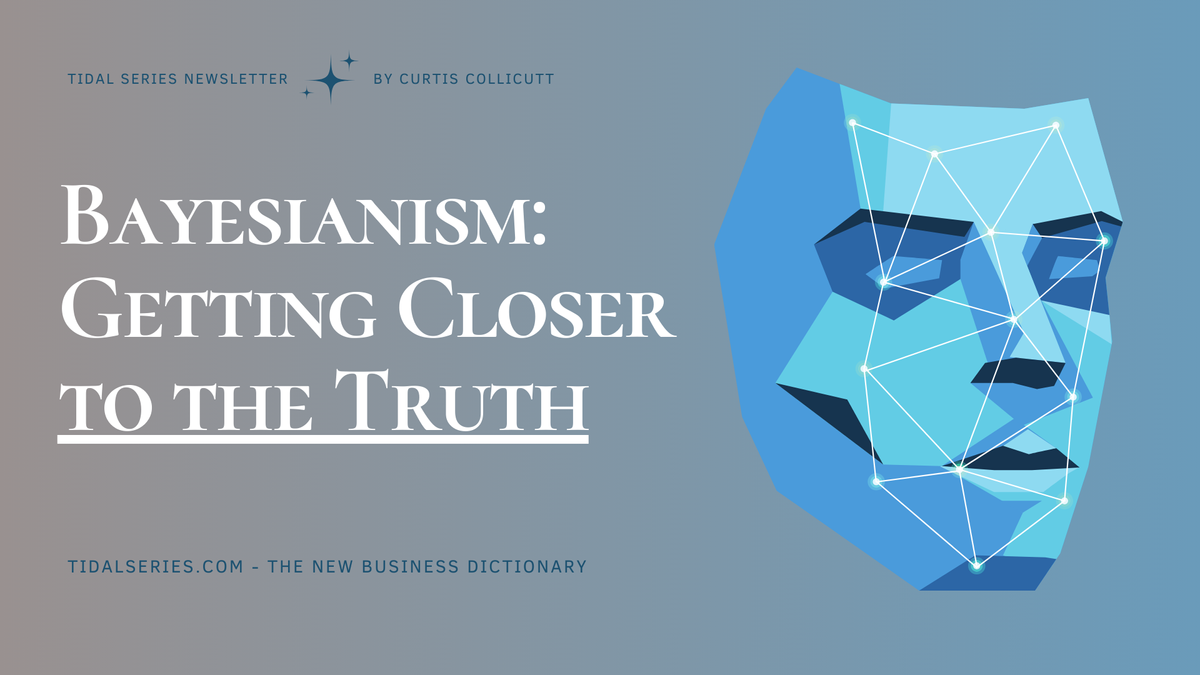
Past performance is not necessarily indicative of future results. - Every ETF and Mutual Fund Ever
Frequentist statistics tries to eliminate uncertainty by providing estimates. Bayesian statistics tries to preserve and refine uncertainty by adjusting individual beliefs in light of new evidence. [4]
This is part of a series of posts on The New Business Dictionary.
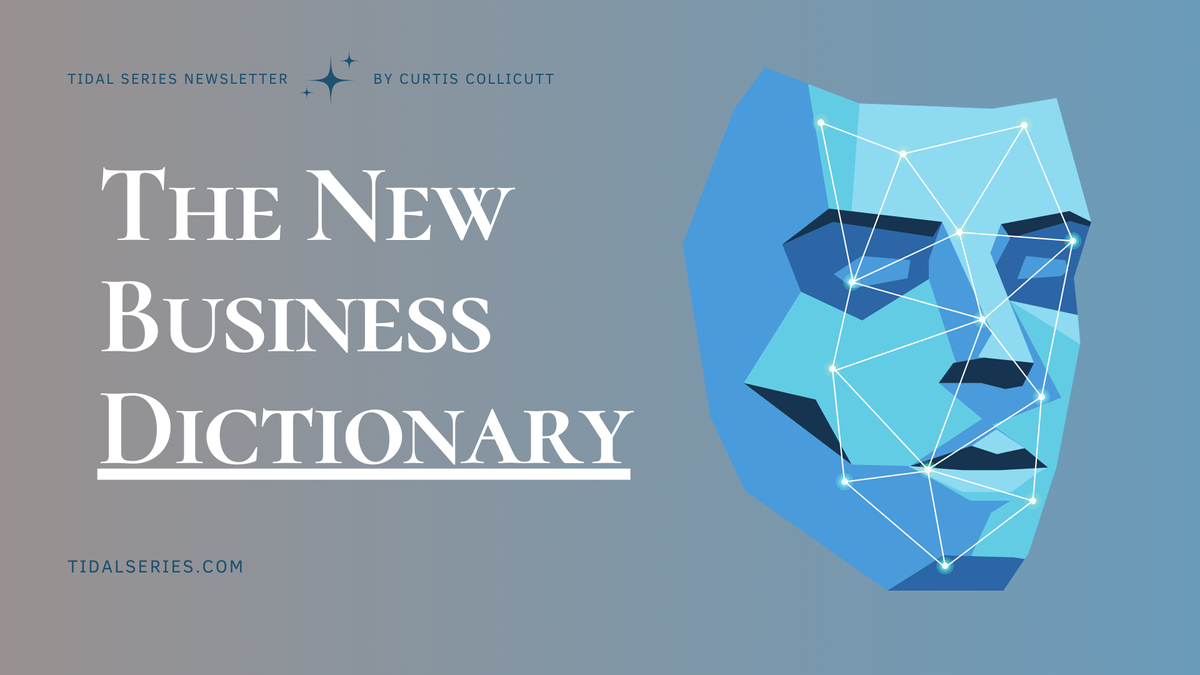
Updating Your Priors
Building new businesses is extremely difficult. It's an arduous trek, uphill forever. Probably too difficult for our economic systems; we should all be finding ways to make building a new business easier, cheaper, and faster. But I digress.
How do people make good new business decisions? Most of the time it is based on gut instinct and intuition, and a healthy dose of "never giving up." But that can only take you so far. Not everyone succeeds. What's more, those of us who look for ideas from afar end up seeing a handful of high-profile new business winners, creating a survivorship bias that skews our understanding. Could there be another way to make smart decisions about new ventures?
This overview will not attempt to perfectly or precisely define Bayesian and all its related terms, as that is beyond the scope of a single newsletter entry. Instead, let's start with (hopefully) enough information to understand how business professionals might use these terms in real-world practice, whether the use is right or wrong. In the "Further Reading" section, I'll provide links that could help you learn more about Bayesian thinking.
Thomas Bayes
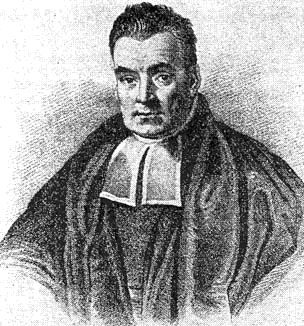
The Bayes theorem is named after, you guessed it, Thomas Bayes. [3]
It's probably not polite to boil a person's life down to a single sentence, but I'll do it anyway. What I find interesting about Mr. Bayes life is that he died before his important theorem was published by his friend, a Mr. Price. Bayes would never have known, even remotely, that what he had written, what he had discovered, would have such a far-reaching and long-lasting effect, or actually, any effect at all.
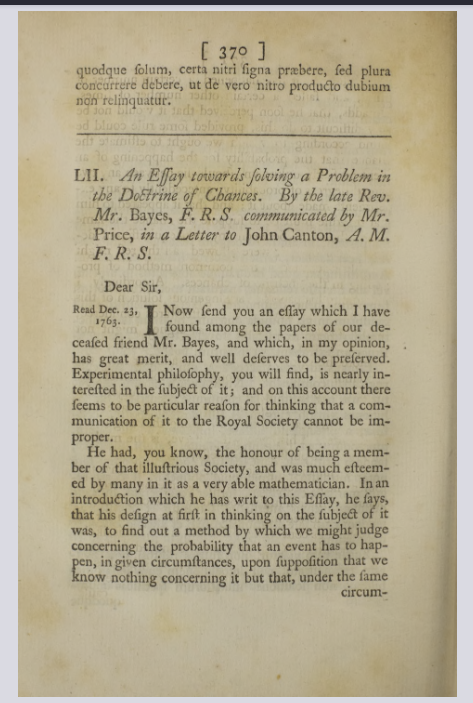
Bayesian Reasoning/Inference
The Frequentist approach is a traditional way of looking at statistics based on how often things happen in repeated experiments. It relies on the long-term behavior of data, assuming that probabilities are related to the frequencies of events in repeated trials. [1]
I think many of us have our own internal definition of what "statistics" and "probability" are, but we don't have the definition quite right, which is why the introductory Statistics 101 course most people take in university is often so difficult.
If we flip a coin 100 or 1000 or (n) times, and about half of them come up heads and the other half tails, we might think that the probability of heads or tails is about 50%, and we can use that information going forward to make decisions, bets, and guesses. To a lot of us, that is simply what probability is, which turns out to be the frequentist or classical method.
Frequentist: "If we repeated this experiment infinitely, what percentage would show this result?"
Frequentist approaches are typically used with large data sets and simple questions. An insurance company would use frequentist methods when analyzing decades of accidents to calculate premiums.
Bayesian, on the other hand, deals with situations where we know less about the systems we're studying and may not have as much data to incorporate prior knowledge.
Bayesian: "Given what we already know and the evidence we've just observed, how should we update our beliefs about this situation?"
Bayesian Definitions
Current/Prior Belief
What do we currently believe about this situation before seeing any new data?
This is your initial probability estimate before taking the new evidence into consideration. In the context of running a business, this might be your expectations around customer behavior based on previous market research. It may be your "gut instinct" which needs updates.
Updated/Posterior Belief
Given everything we now know, what's the probability that [outcome] falls within [specific range]?
This is your new probability, based on considering the new information and updating your prior beliefs. Each new posterior belief becomes the basis for the next round of evidence-based updates, creating a perpetual cycle of updates that hopefully makes our beliefs get closer and closer to the truth, more and more accurate.
Updating Your Priors / Bayesian update / Belief Revision
What should we believe now that wasn't true before we saw this new data?
This is the act of revising your beliefs based on new information. This is ultimately the core of Bayesianism, in that it provides a structured framework for learning from experience rather than clinging to outdated assumptions.
Evidence
The new data or observations that provide the ability to update our priors. We have to ask how good the evidence is, is it relevant, was it collected properly and methodically, is it substantial? Much more than gut feelings.
Bayesian Credible or Confidence Interval
If we were to repeat this measurement process many times, in what range would we expect the true value to fall [percentage] of the time?
This is a statistical range that shows the probability of a true value falling within it, based on observed data and prior knowledge. An example might be when a startup is trying to estimate customer acquisition costs, where we end up with a statement like "There is a 95% probability that your cost per customer will be between $25-28". This would allow startups to make informed business decisions.
Useful in New Business
New businesses, startups, etc. operate in an environment of extreme uncertainty. They are fundamentally challenged by not knowing exactly what will work and what won't. While established companies have long-term revenue streams that are relatively easy to understand and analyze, new businesses, by definition, do not. They don't have the data.
The business world is constantly changing, flipping, bending, and breaking as it works its way through paradigm shifts such as mobile technology, cloud computing, and artificial intelligence, to name just a few technology-related changes, creating opportunities for new businesses that can adapt more quickly. But this is often a disadvantage from a predictability standpoint, which is part of the reason why creating a successful new business is so difficult. But incorporating new information through a Bayesian model can be helpful.
New companies simply don't have enough historical data to rely on the frequentist approach. So, consciously or not, they try to use something closer to Bayesian thinking to make decisions. Maybe it would be valuable to lean into what Bayesian is in terms of building a successful new business, rather than just going with gut instinct and intuition.
Conclusion
This is a brief introduction to some of the terms used in the Bayesian world. In future posts, we'll try to go deeper into how this can help new businesses make decisions.
Further Reading
[1]: https://www.invespcro.com/blog/bayesian-vs-frequentist-ab-testing-which-testing-method-is-better/
[2]: https://royalsocietypublishing.org/doi/10.1098/rstl.1763.0053
[3]: https://en.wikipedia.org/wiki/Thomas_Bayes
[4]: https://www.quantstart.com/articles/Bayesian-Statistics-A-Beginners-Guide/